Herat Joshi, Data Science Lead at Great River Health, highlights how ensemble AI models improve early breast cancer detection by boosting accuracy and reducing invasive diagnostics, marking a major advance in precision medicine.
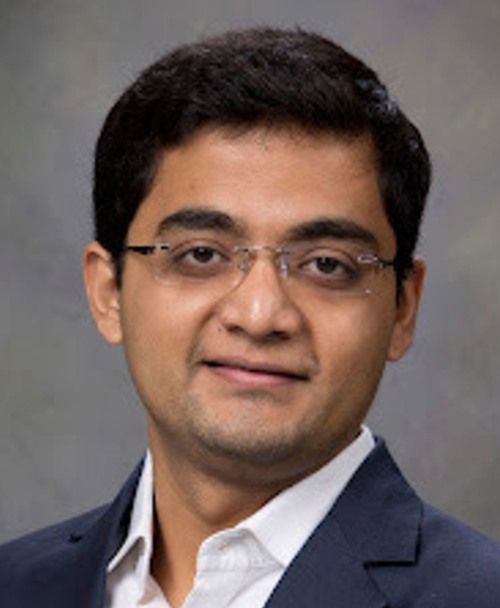
Breast cancer remains one of the leading causes of cancer-related deaths worldwide. According to the World Health Organization (WHO), breast cancer is the most common cancer among women globally. Recent data indicates that approximately one in eight women (12.5%) worldwide will be diagnosed with breast cancer in their lifetime, highlighting the critical need for early detection and accurate diagnosis. Traditional methods, while effective, often face limitations, including high rates of false positives and negatives, class imbalances in data and the invasive nature of some diagnostic procedures. With the increasing availability of large datasets and advancements in Artificial Intelligence (AI), the medical community has begun turning to Machine Learning (ML) as a powerful tool to enhance diagnostic precision and improve patient outcomes. Among these advancements, ensemble AI techniques represent a significant leap forward in breast cancer detection, offering a more reliable and accurate method to identify malignant tumours at an earlier stage.
Challenges in breast cancer detection
Diagnosing breast cancer is fraught with challenges. One of the biggest issues is class imbalance in breast cancer data. This occurs when most cases are benign, making it harder for algorithms to identify malignant cases accurately. This imbalance can lead to false negatives—where cancer is missed—or false positives, subjecting patients to unnecessary procedures and stress.
Timeliness is another concern. Early detection is crucial to improving survival rates, but traditional diagnostic methods can be slow, delaying treatment and reducing the chances of a better outcome.
The power of ensemble Machine Learning models
Ensemble Machine Learning models work like a panel of experts collaborating to solve a problem. Instead of relying on a single model’s prediction, ensemble models combine the insights of several models, resulting in a more accurate and reliable diagnosis.
In my research on improving diagnostic tools for women’s health, I developed an ensemble model that incorporates multiple Machine Learning techniques, including CatBoost, XGBoost, Random Forest and Linear Discriminant Analysis (LDA). This combination resulted in an impressive classification accuracy of 98.83%, significantly better than any individual model’s performance.
Each model offers unique strengths: one excels at processing complex health data, another detects hidden patterns, while others reduce variability and improve differentiation between health conditions. Together, they form a robust tool for detecting breast cancer and advancing personalised care in women’s health.
Addressing the issue of class imbalance
Class imbalance, where malignant cases are outnumbered by benign ones, is a significant challenge in breast cancer diagnosis. By using advanced techniques like resampling and synthetic data generation (such as SMOTE), ensemble models can ensure that both malignant and benign cases are properly represented. This reduces the risk of missing a cancer diagnosis and improves the overall accuracy of early detection.
Impact in clinical settings
The potential impact of ensemble AI techniques in clinical settings is profound, particularly in reducing the need for invasive diagnostic procedures like biopsies. Although biopsies are still the gold standard for confirming cancer, they can be invasive, costly and cause discomfort for patients. AI-powered diagnostic tools, particularly ensemble models, offer the potential to reduce unnecessary biopsies by accurately classifying tumours based on non-invasive imaging data.
While AI cannot fully replace biopsies, it can help identify cases where they may not be needed, thereby sparing some patients from undergoing this procedure. Moreover, these tools enhance early detection, allowing physicians to implement treatment plans sooner, which can improve outcomes—especially in breast cancer, where early intervention is closely linked to higher survival rates. The ability of AI to quickly analyse vast amounts of data can also expedite the diagnostic process, leading to more timely and effective treatments.
AI in personalised breast cancer treatment
Beyond diagnostics, ensemble AI models offer immense potential for personalised breast cancer treatment. These models allow for a deeper understanding of each patient’s unique cancer profile, taking into account factors like genetic predisposition, tumour characteristics and responses to previous treatments. This level of insight enables physicians to tailor treatment plans to the individual rather than applying a one-size-fits-all approach.
For instance, AI can help identify which patients are likely to benefit from specific treatments, such as hormone therapy or targeted therapies like HER2 inhibitors. By predicting the likelihood of success for various treatment options, AI helps physicians make better-informed decisions, ultimately improving patient outcomes and minimising the risk of over- or under-treatment.
Moreover, as more data becomes available, AI-powered models continue to learn and adapt, further refining treatment recommendations over time. This adaptability is crucial in oncology, where treatments are constantly evolving.
Conclusion: A bright future with AI and FemTech
Ensemble AI techniques are driving a new era of precision medicine in breast cancer detection and treatment. While these models have limitations—such as their reliance on high-quality data and the expertise of clinicians—they are valuable tools that complement existing methods, reducing unnecessary procedures and enhancing patient outcomes.
As AI continues to evolve, its true potential will be unlocked through collaboration between healthcare professionals, researchers and AI developers. This partnership will be essential in overcoming challenges and driving innovation. The future of women’s health is on the brink of transformation, with AI playing a pivotal role in delivering faster, more accurate diagnoses and ultimately saving lives.